PhD Thesis
Personalising robot assistance for cognitive training therapy
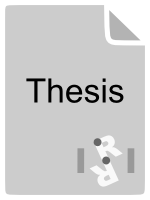
Student/s
Supervisor/s
Information
- Started: 01/03/2017
- Finished: 13/10/2022
Docs
Description
There are 50 million people worldwide living with dementia, and this number is projected to triple by 2050. While there are no pharmacological treatments for this syndrome, behavioural therapy such as cognitive training can maintain or improve the patients' general cognitive functioning, eventually prolonging ageing in place. However, the number of patients who require such treatment greatly exceeds the number of healthcare professionals' availability.
Robots have the potential to bridge this gap by facilitating this training regimen and enhancing therapists’ effectiveness in delivering cognitive therapy. A key enabling technology is personalisation. Indeed, the ability to personalise such treatments to suit the preferences and goals of an individual is crucial to improving the quality and efficacy of the intervention and increasing users’ engagement over long-term interactions. Despite the recent advances, many questions still need to be addressed regarding the design, development, and deployment of social robots for such purposes.
This thesis postulates a set of these questions with which we aim to pursue our main goal: the development of a fully autonomous robot for delivering cognitive training. In the scope of this thesis, we tackled personalisation by designing robots that are both adaptable and adaptive. Adaptable in such a manner as to enable therapists to set them up and customise their high-level behaviour. Adaptive in such a manner that they can tailor their intervention to suit the individual’s unique needs as they evolve through time. Building upon this idea, the work has taken a therapist-oriented approach in which healthcare professionals’ expertise can be reflected in the design of a set of functionalities needed for the development and deployment of a personalised fully autonomous robotic system. Toward such a goal, this thesis explores the impact of robots' human-like characteristics, such as personality, communication style, and backchannelling behaviour, on the users’ intention to use the robot and their task performance. On the other hand, it investigates several AI techniques for robots' reasoning, aiming at learning socially assistive behaviour that better suits the user's abilities.
This thesis culminates by proposing a novel framework called aCtive leARning agEnt aSsiStive bEhaviouR (CARESSER). CARESSER enables therapists to customise the robot’s assistive behaviour (adaptable) and allows the robot to learn in situ personalised patient-specific policies (adaptive) in a fully autonomous fashion. Through the development and evaluation of CARESSER, this work contributes to both the fields of HRI and AI, especially on how social robots can learn by leveraging therapists' expertise and demonstrations, which has the benefits of speeding up the learning process, eliminating the need for the design of complex reward functions, and finally, avoiding undesired states that may have a negative impact on the patients.
The thesis has been awarded the 2023 Georges Giralt PhD Award for the best PhD Thesis in Robotics by the euRobotics.
The thesis has been awarded the 2022 AIHUB.CSIC prize for the best AI PhD Thesis by the AI Hub of the Spanish National Research Council (CSIC).
En el mundo hay 50 millones de personas que conviven con demencia, y se espera que este número se triplique para 2050. Mientras no existen tratamientos farmacológicos para este síndrome, terapia comportamental como el entrenamiento cognitivo, puede mantener o mejorar el funcionamiento cognitivo general de los pacientes, eventualmente, prolongando el envejecimiento en casa. Sin embargo, el número de pacientes que requieren tal tratamiento excede en gran medida el número de profesionales médicos disponibles. Los robots tienen el potencial de cerrar esta brecha, facilitando este tipo de entrenamiento y mejorando la efectividad de los terapeutas en su tarea de terapia cognitiva.
Una tecnología habilitadora clave es la personalización. De hecho, la habilidad de personalizar tales tratamientos para adaptarse a las preferencias y objetivos de un individuo, es crucial para mejorar la calidad y eficacia de la intervención e incrementar el compromiso de los usuarios durante interacciones a largo plazo. A pesar de los recientes avances, muchas cuestiones acerca del diseño, desarrollo y despliegue de robots sociales para esos propósitos necesitan ser tratadas todavía.
Esta tesis postula un conjunto de estas cuestiones con las cuales intentamos perseguir nuestro objetivo principal: el desarrollo de un robot completamente autónomo para proporcionar entrenamiento cognitivo. En el ámbito de esta tesis, abordamos la personalización diseñando robots que son adaptables y adaptativos. Adaptables de manera que permitan a los terapeutas configurarlos y personalizar su comportamiento de alto nivel. Adaptativos de manera que ellos mismos puedan confeccionar su intervención para adaptarse a las necesidades únicas del individuo a medida que éstas cambian a lo largo del tiempo.
Construyendo sobre esta idea, el trabajo ha tomado una dirección orientada al terapeuta, en la que la experiencia de los profesionales de asistencia médica puede ser reflejada en el diseño de un conjunto de funcionalidades que se necesitan para el desarrollo y el despliegue de un sistema robótico completamente autónomo. Hacia ese objetivo, esta tesis explora el impacto de características robóticas parecidas a las humanas, como la personalidad, el estilo de comunicación, y canalización del comportamiento, en la intención de los usuarios de utilizar el robot y en su rendimiento en la tarea. Por otro lado, la tesis investiga diversas técnicas de Inteligencia Artificial para el razonamiento robótico, intentando aprender el comportamiento socialmente asistivo que mejor se adapta a las habilidades del usuario.
Esta tesis culmina proponiendo una infraestructura novedosa llamada "aCtive leARning agEnt aSsiStive bEhaviouR (CARESSER)". CARESSER capacita a los terapeutas a personalizar el comportamiento asistente del robot (adaptable), y permite al robot aprender reglas personalizadas específicas para cada paciente "in situ" (adaptativo). Durante el desarrollo y evaluación de CARESSER, este trabajo contribuye a los campos de interacción entre humano y robot y el de la inteligencia artificial. Especialmente en cómo los robots sociales pueden aprender haciendo uso de la experiencia y las demostraciones de los terapeutas, que tiene los beneficios de acelerar el proceso de aprendizaje, eliminando la necesidad de diseñar complejas funciones de recompensa, y finalmente, evitando estados indeseados que puedan tener un impacto negativo en los pacientes.
La tesis ha sido premiada con el premio Georges Giralt a la mejor Tesis Doctoral en Robotica por el euRobotics euRobotics.
La tesis ha sido premiada con el premio AIHUB.CSIC 2022 a la mejor Tesis Doctoral en IA por el AI Hub del Consejo Superior de Investigaciones Científicas (CSIC).
The work is under the scope of the following projects:
Follow us!