Research Project
IPALM: Interactive Perception-Action-Learning for Modelling Objects
Type
European Project
Start Date
01/10/2019
End Date
31/12/2022
Project Code
PCI2019-103386
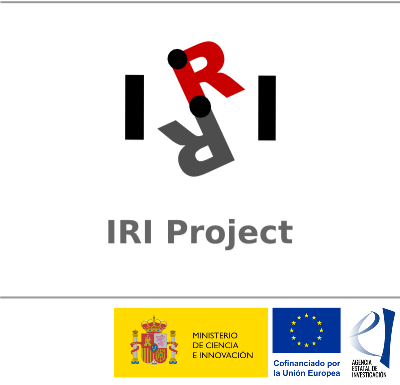
Staff
-
-
Sanchez, Jordi
Researcher
-
Dimiccoli, Mariella
Researcher
-
Agudo, Antonio
Researcher
-
Ren, Ruijie
Support
-
Riba, Edgar
Member
-
Ordoñez, Daniel Felipe
Member
-
Pérez, Alejandro
Member
-
Dhamanaskar, Ameya
Member
Project Description
Project PCI2019-103386 funded by MCIN/ AEI /10.13039/501100011033 and by the "European Union"
In IPALM, we will develop methods for the automatic digitization of objects and their physical properties by exploratory manipulations. These methods will be used to build a large collection of object models required for realistic grasping and manipulation experiments in robotics. Household objects such as tools, kitchenware, clothes, and food items are in focus of many potential applications of robotics, however they still pose great challenges for robot object perception and manipulation in realistic scenarios. We therefore propose to advance the state of the art by considering household objects that can be deformable, articulated, interactive, specular or transparent, or without a strict geometry such as cloth and food items. Our methods will learn the physical properties essential for perception and grasping simultaneously from different modalities: vision, touch, audio as well as text documents. These properties will include: 3D model, surface properties such as friction and texture, elasticity, weight, size, together with the grasping techniques for intended use. At the core of our approach will be a two-level modelling, where a category level model will provide priors for capturing instance level attributes of specific objects. We will build the category-level prior models by exploiting online available resources. A perception-action-learning loop will then use the robots vision, audio, and tactile senses to model instance-level object properties, guided by the more general category-level model. In return, knowledge acquired from a new instance will be used to improve the category-level knowledge. Our approach will allow us to efficiently create a large database of models for objects of diverse types, which will be suitable for example for training neural network based methods or enhancing existing simulators. We will propose a benchmark and evaluation metrics for object manipulation, to enable comparisons of results generated with various robotics platforms on our database.
Consortium:
Imperial College London, United Kingdom (Coordinator)
University of Bordeaux, France
Institut de Robòtica i Informàtica Industrial (IRI), CSIC-UPC, Spain
Aalto University, Finland
Czech Technical University, Czech Republic
Call: European CHIST-ERA 2017
Funds are provided by national research funding organisations. IRI is funded by the Spanish Ministry of Science, Innovation and Universities (MICIU).
Project Publications
Journal Publications
Conference Publications
-
D.F. Ordoñez, M. Martin, A. Agudo and F. Moreno-Noguer. Morphological symmetries in robot learning, 2023 RSS Workshop on Symmetries in Robot Learning, 2023, Daegu (South Korea), pp. 1-5.
Abstract
Info
PDF
-
W. Guo, Y. Du, X. Shen, V. Lepetit, X. Alameda and F. Moreno-Noguer. Back to MLP: A simple baseline for human motion prediction, 2023 IEEE Winter Conference on Applications of Computer Vision, 2023, Waikoloa, Hawaii, pp. 4798-4808.
Abstract
Info
PDF
-
D.F. Ordoñez, M. Martin, A. Agudo and F. Moreno-Noguer. On discrete symmetries of robotics systems: A group-theoretic and data-driven analysis, 2023 Robotics: Science and Systems Conference, 2023, Daegu, Republic of Korea.
Abstract
Info
PDF
-
A. Pérez and A. Agudo. Matching and recovering 3D people from multiple views, 2022 IEEE Winter Conference on Applications of Computer Vision, 2022, Waikoloa, Hawaii, USA, pp. 1184-1193, IEEE.
Abstract
Info
PDF
-
D.F. Ordoñez, A. Agudo, F. Moreno-Noguer and M. Martin. An adaptable approach to learn realistic legged locomotion without examples, 2022 IEEE International Conference on Robotics and Automation, 2022, Philadelphia, Pennsylvania, USA, pp. 4671-4678.
Abstract
Info
PDF
-
M. Dimiccoli, S. Patni, M. Hoffmann and F. Moreno-Noguer. Recognizing object surface material from impact sounds for robot manipulation, 2022 IEEE/RSJ International Conference on Intelligent Robots and Systems, 2022, Kyoto, pp. 9280-9287.
Abstract
Info
PDF
-
J. Shen, A. Agudo, F. Moreno-Noguer and A. Ruiz. Conditional-Flow NeRF: Accurate 3D modelling with reliable uncertainty quantification, 17th European Conference on Computer Vision, 2022, Tel Aviv (Israel), in Computer Vision – ECCV 2022 , Vol 13666 of Lecture Notes in Computer Science, pp. 540-557, 2022.
Abstract
Info
PDF
-
A. Agudo. Piecewise Bézier space: Recovering 3D dynamic motion from video, 28th IEEE International Conference on Image Processing, 2021, Anchorage, AK, USA, pp. 3268-3272.
Abstract
Info
PDF
-
A. Vakhitov, L. Ferraz, A. Agudo and F. Moreno-Noguer. Uncertainty-Aware Camera Pose Estimation from Points and Lines, 2021 IEEE/CVF Conference on Computer Vision and Pattern Recognition, 2021, Nashville, TN, USA (Virtual), pp. 4657-4666, Computer Vision Foundation.
Abstract
Info
PDF
-
J. Sanchez, A. Pumarola and F. Moreno-Noguer. PhysXNet: A customizable approach for learning cloth dynamics on dressed people, 2021 International Conference on 3D Vision, 2021, London, UK (Virtual), pp. 879-888.
Abstract
Info
PDF
-
N. Ugrinovic, A. Ruiz, A. Agudo, A. Sanfeliu and F. Moreno-Noguer. Body size and depth disambiguation in multi-person reconstruction from single images, 2021 International Conference on 3D Vision, 2021, London, UK (Virtual), pp. 53-63.
Abstract
Info
PDF
-
A. Pumarola, E. Corona, G. Pons-Moll and F. Moreno-Noguer. D-NeRF: Neural radiance fields for dynamic scenes, 2021 IEEE/CVF Conference on Computer Vision and Pattern Recognition, 2021, Nashville, TN, USA (Virtual), pp. 10313-10322, Computer Vision Foundation.
Abstract
Info
PDF
-
J. Shen, A. Ruiz, A. Agudo and F. Moreno-Noguer. Stochastic Neural Radiance Fields: Quantifying uncertainty in implicit 3D representations, 2021 International Conference on 3D Vision, 2021, London, UK (Virtual), pp. 972-981.
Abstract
Info
PDF
-
J. Lundell, E. Corona, T. Nguyen Le, F. Verdoja, P. Weinzaepfel, G. Rogez, F. Moreno-Noguer and V. Kyrki. Multi-FinGAN: Generative coarse-to-fine sampling of multi-finger grasps, 2021 IEEE International Conference on Robotics and Automation, 2021, Xian, China, pp. 4495-4501.
Abstract
Info
PDF
-
E. Corona, A. Pumarola, G. Alenyà and F. Moreno-Noguer. Context-aware human motion prediction, 2020 IEEE/CVF Conference on Computer Vision and Pattern Recognition, 2020, Seattle, WA, USA (Virtual), pp. 6990-6999.
Abstract
Info
PDF
-
E. Corona, A. Pumarola, G. Alenyà, F. Moreno-Noguer and G. Rogez. GanHand: Predicting human grasp affordances in multi-object scenes, 2020 IEEE/CVF Conference on Computer Vision and Pattern Recognition, 2020, Seattle, WA, USA (Virtual), pp. 5030-5040.
Abstract
Info
PDF
Follow us!